Businesses and individuals that buy datasets understand that they are getting the raw deal. It is their job to refine it into intelligent insights they can act on. This job is so essential that it is the difference between whether a company succeeds or fails despite using the same data.
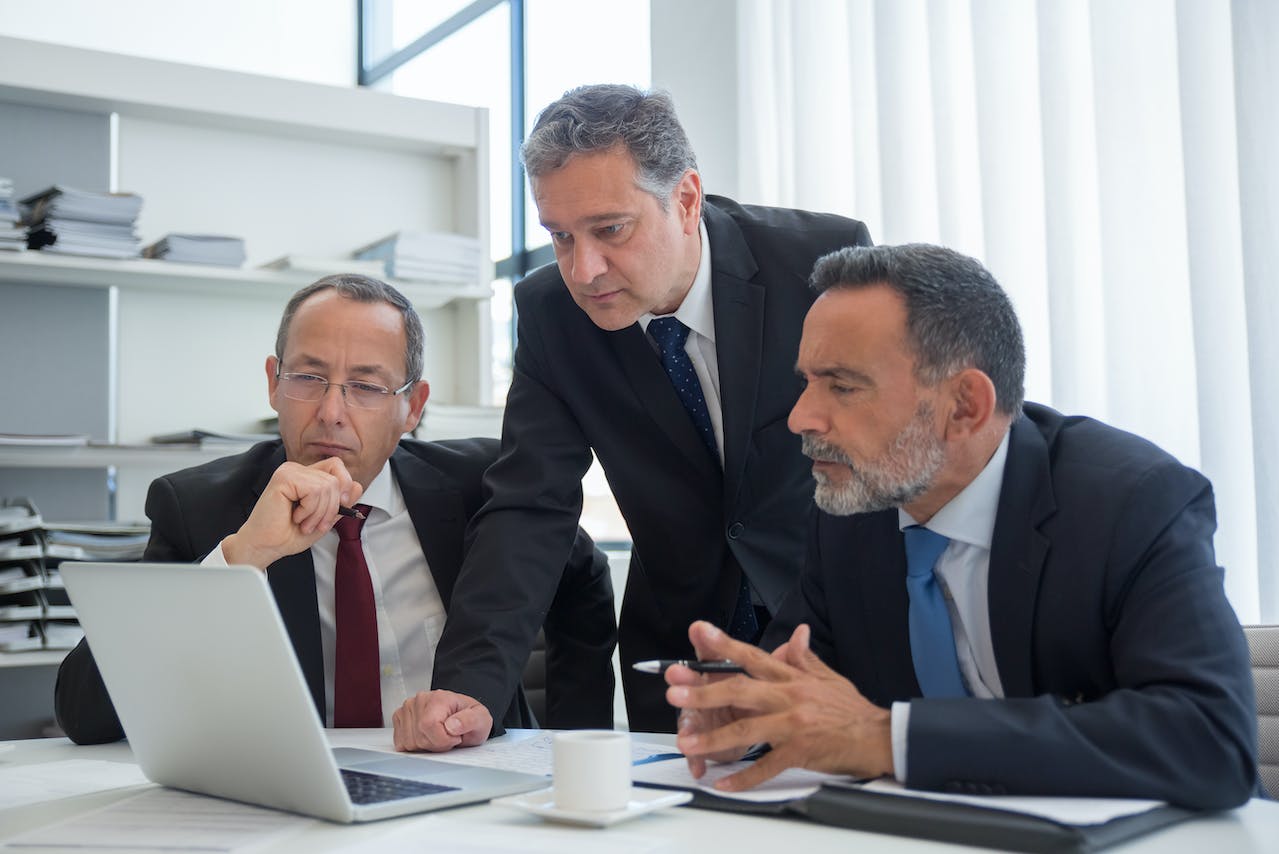
Consequently, businesses allocate multiple times the resources used to buy datasets to derive insight from said data. Read on to learn why datasets are so valuable, how businesses transform them into intelligence, and their use cases in certain industries.
Why are Datasets Valuable to Businesses?
Data helps companies to develop business intelligence and make smarter decisions. But how exactly does this work? Here are some of the ways that datasets contribute to organizational success and create value for businesses:
- Understanding of consumer behavior. Consumer behavior is central to marketing success, and relevant datasets can help businesses understand them. Reliable data sets from social media conversations, retail marketplace buying patterns, customer reviews, etc., offer useful insights. The insights generated can then influence marketing and advertising decisions.
- Facilitation of market analysis. Datasets on the components of a market can provide a comprehensive understanding of situations. When employed right, these datasets can help identify predictive trends, strengths and weaknesses, opportunities, etc. Businesses can then utilize the new knowledge in their overall strategy.
- Improving operational efficiency. Business growth can manifest in various ways, including outward expansion and internal optimization. Companies regularly look inward to identify areas of improvement within their operations. Datasets on employee performance, productivity, and resource allocation can help companies streamline work and improve overall efficiency. Other areas of business operations that could benefit from analyzing relevant datasets include supply chain management, human resources management, reporting hierarchies, etc.
- Personalization of products and services. To improve their performance in international markets, businesses sometimes turn to product/content localization. However, they still require accurate consumer data to help identify preferences and cost-effective ways to adapt. Accurate datasets could help this goal and consequently secure consumer loyalty and larger market shares.
- Planning. Business planning involves thinking long-term. As much as vision plays a part in this, data can provide the foundation to guide the implementation of vision. Companies that buy and curate the right datasets can potentially glimpse the future by analyzing historical and current trends. Such analysis may provide insights into investments to make or avoid in the future.
Transforming Datasets into Actionable Intelligence
The thing about raw data points is that they are meaningless. That is not to say that they make no sense. However, considered individually, they provide very little insight or direction. As such, it is essential that they undergo a process to convert them into intelligent information businesses can act on. The systematic process of this conversation is as follows:
- Data Collection. The first step of the data conversion process is to collect and save it in an accessible format. Sometimes, the collection is from a single source, and sometimes it is from multiple sources. Stream processing deals with data in real-time as it flows through the pipeline. As such, this raw data may be present in different structures and formats.
- Data cleaning. Differing structures and formats can also come with errors and missing values. Thus, dataset curators clean the data, correcting the issues they identify, scaling the variables, and transforming them as needed. In the case of datasets obtained from multiple sources, cleaning would include aggregation into a single dataset.
- Feature engineering. This is a step in the process suitable for developing datasets for machine learning models. Here, the cleaned data undergoes manipulation and transformation into features employable for said learning.
- Data analysis. Datasets intended for analysis, though, would undergo analysis to identify relationships between variables. At this stage, data engineers also employ advanced analytics and models to search for hidden correlations.
- Data visualization. Here, analysts use graphs, plots, charts, dashboards, etc., to present the complex information garnered in an easy-to-understand form.
- Interpretation of data. The same data could mean different things to different people. As such, interpretation is the process of applying relevant business context to data to create insight.
It is the insight generated from the interpretation that businesses incorporate into their decision-making process. When companies buy datasets, they are buying more than just the data; they are buying the process behind it as well.
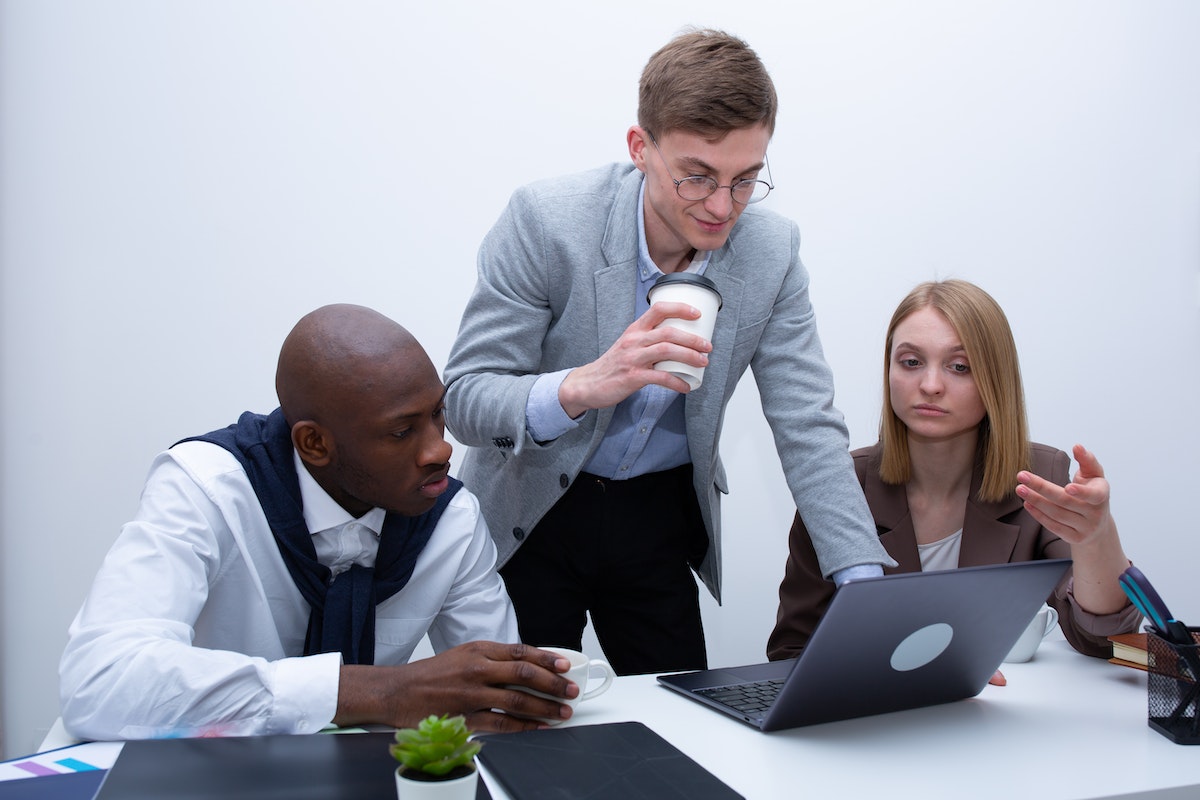
Use Cases of Datasets
Data is a versatile resource used in arguably all industries. As such, its use cases are difficult to quantify. That said, below are some examples of how businesses employ datasets:
- Businesses in industries varying from finance to environmental monitoring use datasets to train machine learning models.
- Researchers in the healthcare industry use datasets spanning years to identify drug-drug interaction, contraindications, adverse effects, varying pharmacological responses, etc.
- Marketers employ datasets to understand consumer behavior, assess campaign performance, identify customer preferences, improve experiences, etc.
- Governments use datasets to inform policy (economic policy, security measures, etc.) and execute projects (urban planning, planning road networks, etc.).
- Interested academic parties utilize datasets to monitor performance, assess teaching habits, conduct research, and personalize learning solutions.
Conclusion
In the context of business intelligence, datasets are seeds. The process of transforming the data helps them bloom into actionable insights. Businesses then use these insights to inform their operations and decisions. The moral lesson here is to not ignore datasets, as they are a powerful resource for growth.