It’s common knowledge that data scientists are high in demand. The profession offers high-income potential and job flexibility and satisfaction – meaning not only are data scientists in high demand, but it’s also an appealing job prospect for many people.
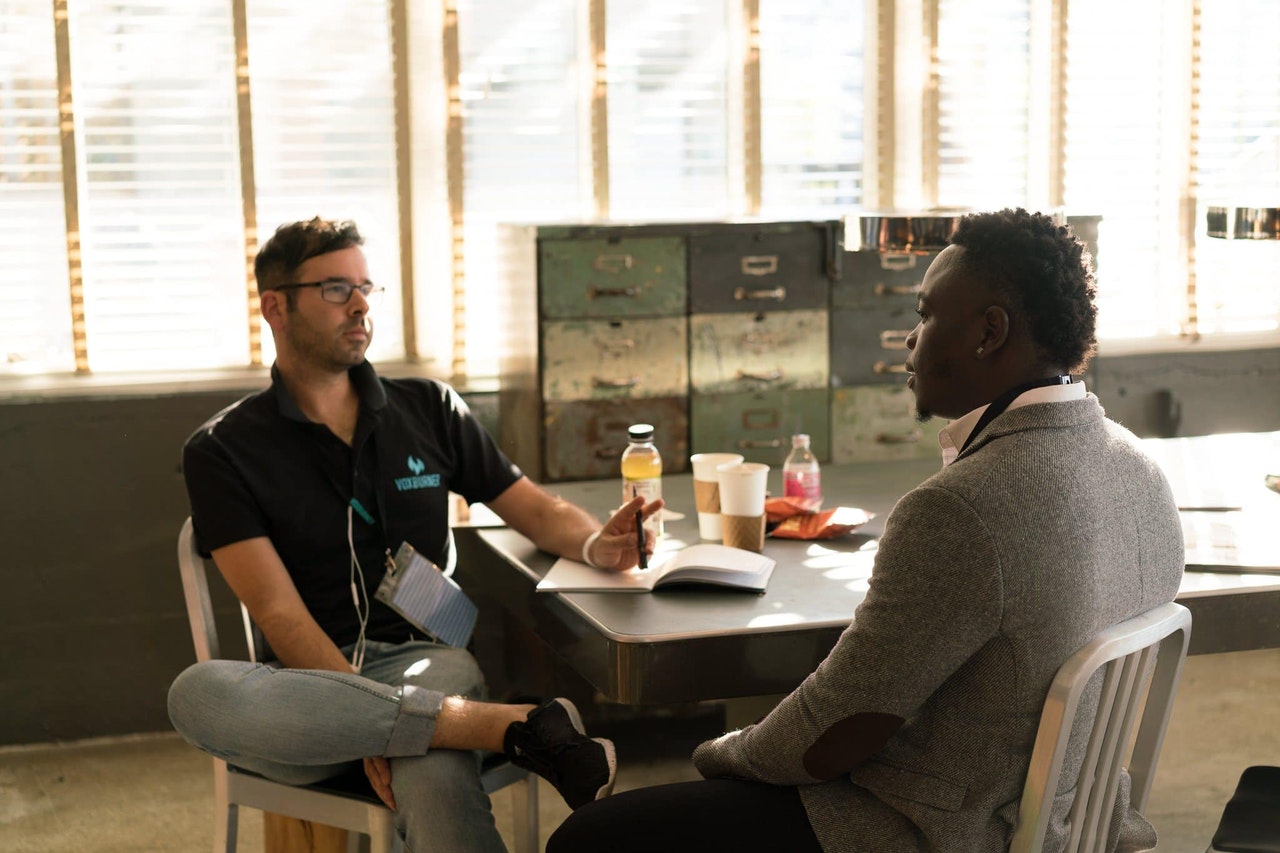
Those who have decided to pursue a career in data science have probably found themselves in a state of disappointment.
While all job hunting is tedious and often difficult – what with constant cover letter writing and endless interviews only to never heard from the company again – it seems particularly difficult for data scientists.
So why is finding a job in data science so hard?
Even though you use a job search site to find opportunities, it seems had to get a response. There are several reasons why you might be struggling to find a job in this – what’s supposed to – high-demand profession. Below are just a few:
Companies don’t know what they need
When it comes to recruitment agencies, as data science is such a new role, most staff aren’t quite sure what they’re supposed to be looking for. Similarly, companies that think they need data analysis aren’t particularly sure what they need and what they should be advertising for.
It can be difficult to know what types of skills the best candidate will have with any new role. Most HR teams or hiring managers aren’t entirely sure what a data scientist is, and what their day-to-day role will look like.
With this in mind, it might be a case of searching for different terms on job sites. Data science jobs may be listed under other roles, so it’s always worth broadening your job search to look at each job in more depth to understand whether it’s right for you.
The interview process is missing the mark
Just like how the recruitment process isn’t clear, the interview process can also miss the mark. Without knowing the proper skill set a data scientist needs, the interviewer can neglect to ask the right questions to find out whether candidates have the right abilities needed for the job.
This can be unfair to the candidates who have more specialized qualifications. So, if you’re struggling to nail the interview process for a job you know you could do well, it could be a case of ‘it’s not you, it’s them…’
The struggle to calculate ROI
When it comes to hiring new staff, it’s all about what the company can afford and how beneficial the new employee will be. As such, companies will be required to calculate the ROI (return on investment).
As with any job, the hiring and training process costs a lot. And the value of a data scientist isn’t immediately provided once they start the job. As such, it can be difficult for businesses to calculate ROI for hiring a data scientist, and as a lot of businesses are impatient, this can significantly impact whether they are open to hiring someone in the first place.
Practical vs. Academic knowledge
While the previous three reasons finding a job is so hard aren’t directly related to you as a candidate, that’s not to say you couldn’t also be the thing that’s stopping you.
Those that have dedicated their time to academics can often lack the practical knowledge required for the working environment.
While the basics will generally stay the same, the world of data science is constantly evolving. There’ll be new findings and different algorithms, meaning a career in data science will require you to update your skills and knowledge constantly.
To set yourself up for success, you might want to consider taking an online Masters in Applied Statistics so that you can understand more about how to apply your academic skills to the practical world. Being able to adapt to different events and showing the hiring team your practical skills for problem-solving could make the difference and gain you your dream job.
You’re struggling to sell yourself
As with any job search, it’s all about how you market yourself to prospective employers. Despite having all the right experience and qualifications, the perfect candidate often slips through the gaps – leaving them feeling like they’re missing something or did something wrong during the interview.
More often than not, highly qualified, and skilled applicants lack the confidence to sell themselves. And sadly, the interview process is all about marketing your talents.
Before heading to your next interview, consider working on your confidence or prepare answers that prove you know your stuff and you’re not afraid to flaunt it. Check out these interview tips for help, too!
Final Thoughts
So, as we all know, finding a job is never easy. It can be nerve-wracking, tiring, and long. Let’s also not forget about the emotional drain it can have: With every rejection letter or for every business that completely ignores your application, your confidence can take a hit. Are you really good enough for this job? Should you be applying for roles at a lower pay grade? All of this doubt can make the process even more upsetting and unenjoyable.
However, it’s worth remembering – especially when it comes to jobs in data science – that you’re not always the issue. You might have all the skills and experience you know you need, but the recruitment agencies don’t know what they’re looking for. Similarly, companies might not understand the need for data science and quickly become impatient when they don’t see a significant ROI quickly. This all makes finding a job more difficult.
So, if you’re struggling to find a job right now, don’t lose hope. Take this time to focus on building your own portfolio and finding opportunities to develop practical skills. You can also take time to work on selling yourself and refining your interviewing skills.
Lastly, you can also try to market yourself and create your own dream job. While some companies don’t realize they need data science, this means there’s the chance to create the job from scratch. All you have to do is believe in yourself and find a way to prove how valuable you will be.
Good luck!