Rapidly changing business modules, shifting political landscapes, changing consumer attitudes, global pandemics, and economic uncertainty have increased in the modern era, forcing businesses and organizations to adopt methods that promise slimmer margins for errors. Owing to such demands, many technologies have developed in recent times. Data analytics is one of the most popular ones, which has become an integral part of the decision-making process and operations. Data analytics mainly helps businesses process and analyze vast amounts of data, also called big data, enabling them to gain insights into their market, customers, and business operations.
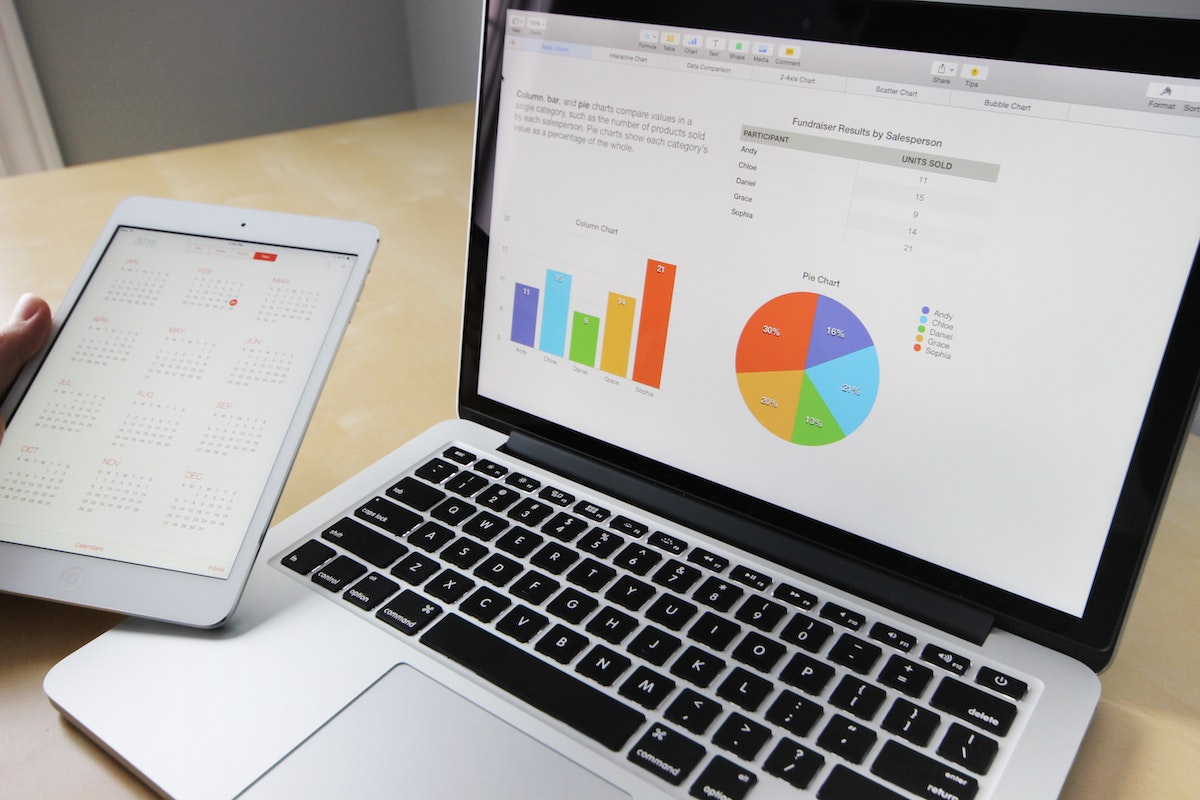
What is Data Analytics?
Today’s businesses grab every opportunity to improvise their existence and service in the global market. Companies that wish to reach higher positions often try to beat the odds by making smarter choices in technology. In the modern era, businesses and multinational organizations are investing in data analytics methods and techniques to collect as much information as possible and use it to make better and more informed business decisions.
Before making any decision in life, we often research and tick the pros and cons to make smarter choices and decisions. The same theory applies even in the business field. No company can succeed in this competitive market without using well-researched data. This is where data analytics or analysis comes into play. It is mainly the process of cleaning, filtering, and processing raw data to extract actionable information, which helps businesses make further decisions. One of the primary benefits of incorporating data analysis procedures in an organization is to make better decisions based on the collected data. Data analysis is also important for processing big data into usable information.
In order to utilize the full efficiency of the field, data analysts often follow certain methods and techniques, such as the ones mentioned below in this article.
Methods and Techniques of Data Analysis
Regression Analysis
In this method, data analysts understand the dependent and the independent variables to study market trends. The Dependent variable refers to the main variable, which talks about the store figures, sales, etc. In contrast, the independent variable refers to the factors that can affect the dependent variable, like weather conditions, market trends, and demographics. This type of analysis aims to study how every independent factor affects the main variable to draw trends and patterns in a graphical format. There are several types of regression analysis methods, depending on the data under analysis.
Depression Analysis
Depression analysis is a popular diagnostic method that is used to determine how your data is spread across different platforms. It provides information on the variation between different data items, which can help determine the relevancy and reliability of data. The primary step followed in this process is to measure the variations among different data points, note down the values and compare them with the data from the standard deviation in the datasheet. If there is a higher deviation or the data is stretched, it means the depression is high. It is mainly used to assess the investment risk in a particular business.
Neural Network Analysis
Artificial neural network analysis is the most modern data analytics method, which uses machine learning to analyze and predict how the human brain works. These networks recognize patterns and discover trends in the business market. The addition of more data leads to better predictions.
In this method, the data is introduced into the predefined network, which produces more info on market predictions, which is very close to how a human brain functions in similar situations. This data analysis technique is mainly used in the financial sector to study and forecast market outcomes, asses risk management, and analyze transactions.
Grounded Theory Analysis
This is one of the best qualitative data analysis methods, mainly following an inductive research approach. It primarily develops theories based on the collected data instead of following the traditional methods of using confirmatory analysis to establish a hypothesis on the data collected. Instead, the technique employs a systematic set of procedures to collect, analyze, and develop a theory around the collected data.
Sentiment Analysis
Sentiment analysis is also called text analysis. This method provides insights into textual data about how customers feel about a business. The method is used to collect qualitative data for trend searches and patterns that are followed in textual data. Sentiment analysis uses an array of algorithms that are generally associated with different opinions, feelings, and thought patterns of the consumer.
Monte Carlo Simulation
This predictive data analytics method considers a forecast of the likelihood of the range of outcomes from an uncertain event or variable. The primary step of this method is to assign variables in a random manner with the possible and predicted distribution of outcomes.
Once each of the values is provided, the model is run several times until a final decision is reached. Every time the model is run on the system, each variable is assigned a different random number, the results of which are recorded. The likelihood of all the possible outcomes will be provided once the stimulation of the entire process is complete.
Discourse Analysis
It is yet another qualitative analysis method that attempts to understand how the world makes use of business language and jargon. This technique also has multiple approaches, which involve defining the objective, establishing content, analysis of the content, and developing a theory around the content to review the language.
Time Series Analysis
Time series is a statistical analysis method in data analytics that is used to forecast different patterns over a set period. For example, it follows a particular series used to stock up on sales by picking up trends and patterns to make informed business decisions.
Top Data Analytics Tools
Irrespective of the methods and techniques followed in data analytics, there are several tools that can help in the popularity, learning, and performance of business modules.
Python
Python is mostly a scripting language that is easier than any other language. It is mostly used in different data analytics procedures. Since this language has an extensive array of machine learning and artificial intelligence libraries, it is useful in various industries to make informed decisions.
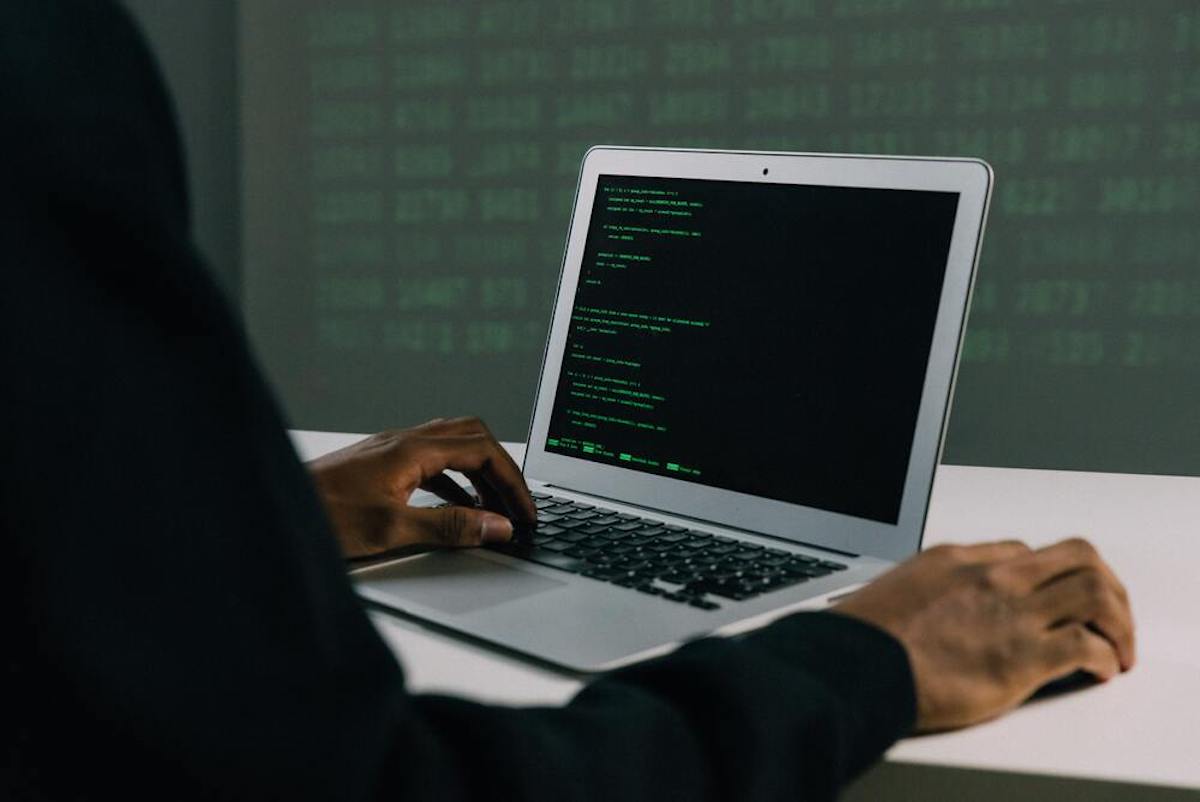
Tableau Public
It is one of the free data visualization tools which helps in linking data from different sources. From the gathered data, it produces dashboards with apt information like maps, real-time changes, and other essential elements required for business growth.
R Programming
R is one of the most popular premium data analytics tools extensively used for data modeling, which helps alter the data in different forms. According to experts, R programming has also outperformed SAS in different data capacities, results, and other species to produce performance analysis results.
Apache Spark
This tool was created in 2009 and helped in large-scale data processing that processes data 100 times more quickly than any other data processing tool in the market. It is also popular for having different machine-learning models and data pipelines. It is also one of the most extensively used tools for various data science procedures like regression, filtering, clustering, collaborative classifications, and so on.
SAS
It is a data manipulation programming tool that creates a dynamic ecosystem for data analytics. In this modern world, it is one of the best tools which is used for profile prospects and clients, along with forecasting actions and improving communications across different business channels.
Importance of Data Analytics
Here are some reasons why data analytics is crucial for today’s businesses.
- Improved customer targeting: The different techniques used in data analytics helps in focusing the customers by rightly guiding to which target group advertising campaigns can be encouraged, hence enhancing customer targeting.
- Provides better problem-solving methods: In the business world, informed decisions are the most successful decisions. The data received by data analytics techniques and methods helps businesses get hold of raw data, which helps them in predicting future challenges and provide necessary solutions to problems that can be faced in the future. This will also help businesses avoid costly pitfalls.
- Reduced operational costs: Data analytics is one of the best ways to know where businesses need to focus their resources. It also provides information on which departments or campaigns should be scaled back or outright eliminated in order to reach business goals.
Conclusion
Data analytics is one of the important concepts in modern business development and a way to help reach business goals. In order to turn the raw data into a more actionable form, it is important to employ different techniques and methods, as mentioned above. Data analytics methods provide insights into your business’s market trends and workings and how businesses can develop different techniques to improve their productivity. Many industries in the modern era have a treasure trove of data that helps them in digital processes to achieve their business goals and turn this data into valuable and meaningful insights for better decision-making. Results from data analytics procedures mainly help businesses in revenue growth, operational performance, building stronger customer relationships, and other essential business sectors.